Big data: The next frontier for innovation
Posted by Armando Brito Mendes | Filed under materiais para profissionais
The amount of data in our world has been exploding, and analyzing large data sets—so-called big data—will become a key basis of competition, underpinning new waves of productivity growth, innovation, and consumer surplus, according to research by MGI and McKinsey’s Business Technology Office. Leaders in every sector will have to grapple with the implications of big data, not just a few data-oriented managers. The increasing volume and detail of information captured by enterprises, the rise of multimedia, social media, and the Internet of Things will fuel exponential growth in data for the foreseeable future.
Tags: big data, data mining, modelos empresariais
What are you going to do with that degree?
Posted by Armando Brito Mendes | Filed under estatística, visualização
Jobs by college major
This is a quick Sankey visualization of how college majors relate to professions, based on data from the American Community survey. On the left are the largest college majors; to the right are the most common professions.
To see broad fields like “Sciences” and “Humanities”, see the edited version of this page.
The width of each stream shows how many people with that major are in that field. (The color shows whether that’s more or fewer people than expected based on how big the major is: hover over to see just how many more it is.) The width of each stream shows how many people with that major are in that field. (The color shows whether that’s more or fewer people than expected based on how big the major is).
You surely see that the lines are too small to understand in most cases: to actually see what’s going on with a particular field or job, click on a box and the chart will filter down to just the people who either majored in the field, or ended up employed in the job. (Click on one of the connecting lines to see both at once.)
I have not developed this that far because I am not sure how useful it ultimately is: my basic goal was a quick way to see, for example, what jobs history majors ended up in. (Largest is lawyers, but also schoolteachers; what you would expect, but worth knowing.)
You might also like my visualization of changing college degrees over time.
Tags: belo, Estat Descritiva
Vector maps on the web with Mapbox GL
Posted by Armando Brito Mendes | Filed under mapas SIG's, materiais para profissionais, software, visualização
Online mapping just got an upgrade:
Announcing Mapbox GL JS — a fast and powerful new system for web maps. Mapbox GL JS is a client-side renderer, so it uses JavaScript and WebGL to dynamically draw data with the speed and smoothness of a video game. Instead of fixing styles and zoom levels at the server level, Mapbox GL puts power in JavaScript, allowing for dynamic styling and freeform interactivity.
For the non-developers: Online maps are typically stored pre-made on a server, in the form of a bunch of image files that are stitched together when you zoom in and out of a map. So developers have to periodically update the image files if they want their base maps to change. It’s a hassle, which is why base maps often look similar. With Mapbox GL, making changes is easier because the development pipeline is shorter.
More details on the JavaScript library here.
Tags: desnvolvimento de software, mapas
Wi-fi revealed
Posted by Armando Brito Mendes | Filed under Sem categoria, visualização
Digital Ethereal is a project that explores wireless, making what’s typically invisible visible and tangible. In the piece above, a handheld sensor is used to detect the strength of Wi-Fi signal from a personal hotspot. A person waves the sensor around the area, and long-exposure photography captures the patterns.
Reminds me of the Immaterials project from a while back, which used a light stick to represent signal strength rather than a signal light.
Tags: belo
European Commissioner for the Digital Agenda Neelie Kroes Speeches
Posted by Armando Brito Mendes | Filed under materiais para profissionais
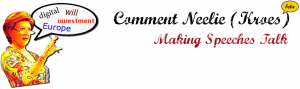
Os discursos da Neelie seguem as tendências do mercado, pelo q se fala muito em analytics, big data, etc.
Politicians’ speeches are important for shaping the policy debate, but they are too often designed as one-way messages.
We want to open up conversations around them, by making speeches commentable phrase by phrase.
Where best to start than from the European Commissioner for the Digital Agenda, Neelie Kroes?
So just select a speech below and click on the phrases that you want to comment.
Tags: análise de sistemas, modelos empresariais
Tutorial: How to detect spurious correlations
Posted by Armando Brito Mendes | Filed under estatística, materiais ensino
Tutorial: How to detect spurious correlations, and how to find the real ones
Specifically designed in the context of big data in our research lab, the new and simple strong correlation synthetic metric proposed in this article should be used, whenever you want to check if there is a real association between two variables, especially in large-scale automated data science or machine learning projects. Use this new metric now, to avoid being accused of reckless data science and even being sued for wrongful analytic practice.
Tags: data mining, Estat Descritiva, inferência
Markov Chains explained visually
Posted by Armando Brito Mendes | Filed under Investigação Operacional, matemática, materiais ensino, visualização
Adding on to their series of graphics to explain statistical concepts, Victor Powell and Lewis Lehe use a set of interactives to describe Markov Chains. Even if you already know what Markov Chains are or use them regularly, you can use the full-screen version to enter your own set of transition probabilities. Then let the simulation run.
Tags: grafos, otimização
ontologies and data models
Posted by Armando Brito Mendes | Filed under materiais para profissionais, SAD - DSS
Ontologies versus Data Models
Data models have been with us since Ted Codd described normalization in 1970 and Peter Chen published his paper on entity relationship diagrams in 1976. Ontology as a discipline in philosophy can trace its roots to ancient Greece. As applied to data management, it is much more recent than data modeling and has only appeared in the past few years. But just what is the difference between ontologies and data models? If they are both about data, do they not boil down to the same thing?
Tags: captura de conhecimento, data mining
Income inequality seen in satellite images from Google Earth
Posted by Armando Brito Mendes | Filed under estatística, visualização
Researchers Pengyu Zhua and Yaoqi Zhang noted in their 2008 paper that “the demand for urban forests is elastic with respect to price and highly responsive to changes in income.” Poor neighborhoods tend to have fewer trees and the rate of forestry growth is slower than that of richer neighborhoods.
Tim De Chant of Per Square Mile wondered if this difference could be seen through satellite images in Google Earth. It turns out that you can see the distinct difference in a lot of places. Above, for example, shows two areas in Rio de Janeiro: Rocinha on the left and Zona Sul on the right. Notice the tree-lined streets versus the not so green.
De Chant notes:
It’s easy to see trees as a luxury when a city can barely keep its roads and sewers in working order, but that glosses over the many benefits urban trees provide. They shade houses in the summer, reducing cooling bills. They scrub the air of pollution, especially of the particulate variety, which in many poor neighborhoods is responsible for increased asthma rates and other health problems. They also reduce stress, which has its own health benefits. Large, established trees can even fight crime.
Okay, I don’t now about that last part about fighting crime. Without seeing the data, I think that sounds like a correlation more than anything else, but still. Trees. Good.
Tags: análise de dados, data mining, image mining, mapas
A Programmer’s Guide to Data Mining
Posted by Armando Brito Mendes | Filed under estatística, materiais para profissionais
A guide to practical data mining, collective intelligence, and building recommendation systems by Ron Zacharski.
About This Book
Before you is a tool for learning basic data mining techniques. Most data mining textbooks focus on providing a theoretical foundation for data mining, and as result, may seem notoriously difficult to understand. Don’t get me wrong, the information in those books is extremely important. However, if you are a programmer interested in learning a bit about data mining you might be interested in a beginner’s hands-on guide as a first step. That’s what this book provides.
This guide follows a learn-by-doing approach. Instead of passively reading the book, I encourage you to work through the exercises and experiment with the Python code I provide. I hope you will be actively involved in trying out and programming data mining techniques. The textbook is laid out as a series of small steps that build on each other until, by the time you complete the book, you have laid the foundation for understanding data mining techniques. This book is available for download for free under a Creative Commons license (see link in footer). You are free to share the book, and remix it. Someday I may offer a paper copy, but the online version will always be free.
Table of Contents
This book’s contents are freely available as PDF files. When you click on a chapter title below, you will be taken to a webpage for that chapter. The page contains links for a PDF of that chapter and for any sample Python code and data that chapter requires. Please let me know if you see an error in the book, if some part of the book is confusing, or if you have some other comment. I will use these to revise the chapters.
Chapter 1: Introduction
Finding out what data mining is and what problems it solves. What will you be able to do when you finish this book.
Chapter 2: Get Started with Recommendation Systems
Introduction to social filtering. Basic distance measures including Manhattan distance, Euclidean distance, and Minkowski distance. Pearson Correlation Coefficient. Implementing a basic algorithm in Python.
Chapter 3: Implicit ratings and item-based filtering
A discussion of the types of user ratings we can use. Users can explicitly give ratings (thumbs up, thumbs down, 5 stars, or whatever) or they can rate products implicitly–if they buy an mp3 from Amazon, we can view that purchase as a ‘like’ rating.
Chapter 4: Classification
In previous chapters we used people’s ratings of products to make recommendations. Now we turn to using attributes of the products themselves to make recommendations. This approach is used by Pandora among others.
Chapter 5: Further Explorations in Classification
A discussion on how to evaluate classifiers including 10-fold cross-validation, leave-one-out, and the Kappa statistic. The k Nearest Neighbor algorithm is also introduced.
Chapter 6: Naïve Bayes
An exploration of Naïve Bayes classification methods. Dealing with numerical data using probability density functions.
Chapter 7: Naïve Bayes and unstructured text
This chapter explores how we can use Naïve Bayes to classify unstructured text. Can we classify twitter posts about a movie as to whether the post was a positive review or a negative one?
Chapter 8: Clustering
Clustering – both hierarchical and kmeans clustering.
Tags: data mining, previsão